Preparing for the EU Pay Transparency Directive | Download our E-book for free
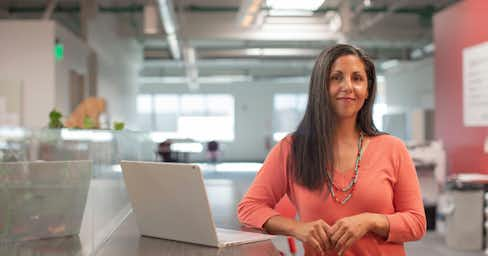
Tech matters: How tech can drive your pay equity journey
The most recent data points to a global pay gap that stands stubbornly at 0.83, meaning that on average, working women only earn 83% of what working men do.
As a result, and as a quick glance at our EU Directive transposition tracker or US laws by state guide will show you, pay equity and pay transparency are becoming increasingly normative and increasingly regulated.
This also means that we’re seeing a boom in people analytics technology. If these tools are well-designed and used effectively, they can increase pay equity as well as make HR professionals’ work more efficient, cost-effective, and reliable.
However, it’s important to choose and use people analytics technology wisely. This article shows you how to avoid two potential pitfalls and how to use technology to increase pay equity and ensure regulatory compliance.
Algorithmic bias: How tech can perpetuate inequity
First, it’s important to understand one major vulnerability of people analytics technology. Many of these tools incorporate machine learning (ML) or artificial intelligence (AI), but ML/AI is only as good as the data that goes into it. If that data reflects old biases, the tool may suggest decisions that reinforce and perpetuate those biases.
For example, an organization might have decades of history in which a disproportionate number of managers were men. Say this organization implements a tool designed to identify the best candidates for management. This tool may “learn” that most successful managers were men, so it will tend to recommend men over women for promotion.
This can lead companies to make a well-intentioned mistake: taking demographic data out of their people analytics tools. They may think that if a people analytics tool can’t identify gender or race, it can’t make biased recommendations. However, demographic characteristics are often correlated with other variables. For example, some demographic minorities may have longstanding ties to specific neighborhoods or towns, making ZIP or postal codes potentially indicative of race or country of origin.
The solution is to include demographic data – while also using bias dashboards and ensuring oversight by knowledgeable human users to prevent biased data from distorting the technology's results. (More on that in a moment.)
Look beyond the outliers
The second common mistake is one we see when companies are actively trying to close their pay gaps. One possible approach to closing such gaps is to focus on the biggest outliers – that is, on the people who are the most underpaid given their experience, performance, etc.
While this may have some effect in the short term, it’s likely that the pay gap will come creeping back over time. This is because the underlying inequities in the organization’s pay structure haven’t been addressed. To make matters worse, focusing only on the outliers causes salary compression in the underpaid group(s).
What to look for in a technology tool
Dashboards to help safeguard from bias
We recommend choosing people analytics technology that has a bias dashboard. This dashboard will show you how your people analytics tool is working for various demographic groups. For instance, some hiring support tools show a demographic breakdown of who looked at a job posting versus who actually applied. This helps HR professionals create job descriptions that avoid discouraging women or demographic minorities from applying.
Even though we often want AI/ML to automate as much as possible, we note that human involvement is still necessary in this process. HR professionals need to understand the data that their tech tools are using and where it might be influenced by systemic bias. They should also be able to review their organization’s data and spot where implicit bias might be getting into the decision-making processes.
Pay equity analysis capabilities
If all that a tool does is identify the outliers in your pay structure, it isn’t doing enough to really help you on your pay equity journey. We advocate using a structured approach in which organizations both address systemic bias and find and remediate inequity in their pay structure.
Your technology for pay equity, then, needs to be able to produce an in-depth pay equity analysis. It should be capable of telling you how different factors contribute to the pay of each group and pinpoint the sources of inequity. For instance, an organization might find that many women in the sales department are making slightly less than expected given their tenure, performance, etc., and that is a major driver of the gender pay gap.
Tailored to your pay equity goals and motivations
Closing a demographic pay gap is never a one-size-fits-all process. Some organizations may be more limited than others in terms of their ability to provide the salary adjustments necessary to close the gap. Where one organization might be able to do it within a few months, others may take several years, parceling the adjustments out carefully over time.
One of the driving factors of these differences is the organization’s motivation for closing its pay gap. Many are primarily motivated by a concern for regulatory compliance; these can be said to be acting “defensively.” These organizations may not need to close their pay gaps all the way to zero. For instance, the EU Pay Transparency Directive requires companies to bring their pay gaps below 5%.
Meanwhile, other organizations want to publicly claim that they have no pay gap, or they may be pursuing third-party fair pay certification. These companies are said to be taking an offensive stance. Even if their pay gap is already relatively small and statistically insignificant, they opt to close it even further.
When working with pay equity technology, then, both types of companies need to make sure that their tools can consider the organization’s budget, maximizing impact while minimizing cost. Good technology will be adjustable and customizable (e.g., providing recommendations for closing the pay gap in two years instead of right this minute). It will also provide statistical significance measures surrounding the pay gap.
Further support with PayAnalytics by beqom
PayAnalytics by beqom was thoughtfully designed by data scientists. This means that our pay equity analysis is accurate and thorough. It lets users determine employees’ pay, pinpoint where biases are, and gain clearer insight into the pay structure.
Because we recognize that there are no one-size-fits all solutions for pay equity, PayAnalytics by beqom is customizable. Users can specify the budget and time horizon for closing a pay gap, maximum raise amount, and more. And whether you are pursuing pay equity to comply with the law or to bring your pay gaps to zero and earn fair pay certification, our software solution has a multitude of reports (both for global or local laws) that you can generate with just a few clicks.
Regardless of whether you have been on your pay equity journey for a while or are just getting started, PayAnalytics by beqom can help. We would love to talk about your motions and goals and how we can support you. Book a one-on-one conversation or software demo today!